Agriculture 5.0: Combating Weeds Using Machine Learning and Robotics
Andrei Mihai
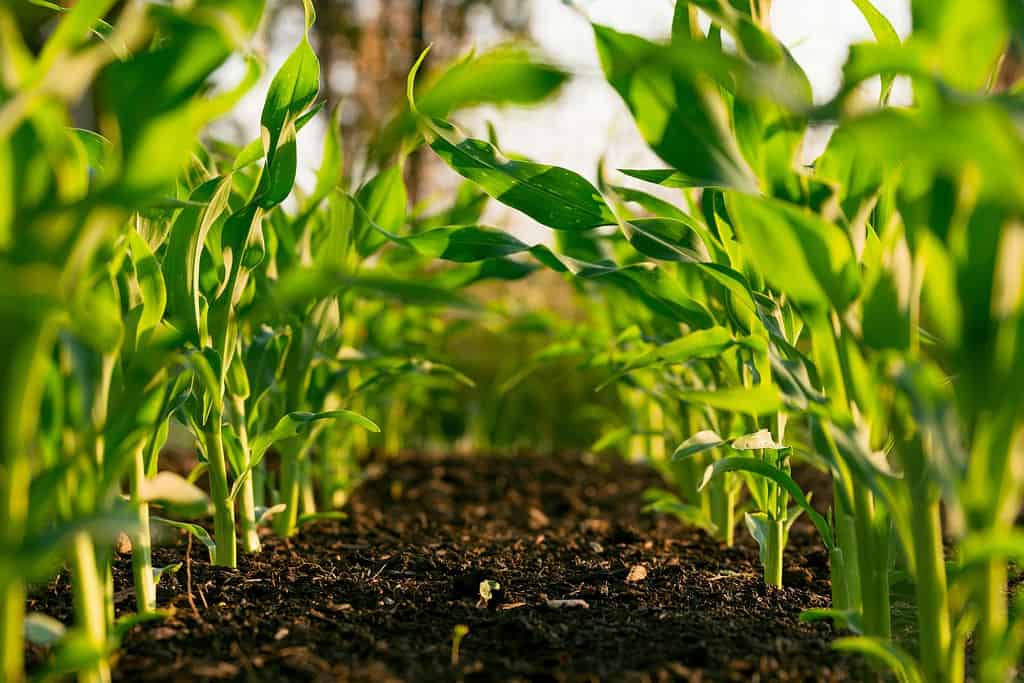
Making agriculture sustainable is one of the biggest challenges of our time. Not only does agriculture generate almost a third of our total greenhouse gas emissions, it uses up enormous amounts of water and fertilizer and contributes to soil erosion and degradation. At the same time, the planet’s population continues to grow, meaning agricultural practices must keep up with that as well. Thankfully, promising new technologies are coming in to help.
The integration of such new technologies is sometimes referred to as Agriculture 5.0, and it represents the latest paradigm shift in agriculture, integrating elements such as machine learning (ML), the Internet of Things (IoT), and robotics.
We have previously written about how the IoT and ML can help with agriculture and in fact, several young researchers who have attended the Heidelberg Laureate Forum (HLF) work in this field. However, the range of applications for this cutting-edge technology is extensive. Cheap sensors can help monitor things like water content and fertilizer usage, thus optimizing resource usage and reducing waste; smart algorithms can detect pests or health problems; and then, there are the weed robots.
Weeds vs. Robots
It is remarkable how well agriculture, one of the oldest practices (arguably a defining one for our species), and cutting-edge technology work together so well. Take, for instance, weed control. Weeds have been one of the biggest challenges for farmers for thousands of years, and are also one of the main focuses of Agriculture 5.0.
In case you have lost track of your “agricultures”, Agriculture 4.0, also known as precision farming, introduced data-driven approaches to optimize agricultural practices. It focused on using telematics, remote data, and precision agriculture to enhance crop quality and yield. However, Agriculture 5.0 goes beyond data-driven practices to incorporate autonomous and unmanned technologies. In Agriculture 5.0, robots and AI play a central role, facilitating tasks such as soil analysis, crop monitoring, and weed management. This terminology is not ubiquitously accepted, but it has become commonplace in the scientific literature as it makes it easier to know the range of technologies and approaches that are most used.
However, in all agriculture iterations, however you may call them, weeds and pests have always been a problem.
Traditional weed management methods, such as the uniform application of herbicides, are often inefficient and environmentally harmful. These approaches can lead to the overuse of chemicals in some areas and under-treating in others. The excessive use of herbicides can harm non-target plants, degrade soil health, contaminate water sources, and contribute to the development of herbicide-resistant weed species.
This is where the new robots come in.
The approach of using machine-learning robots to identify and deal with weeds has already been discussed several times in peer-reviewed research. The “dealing with” part is relatively easy: Either a laser that zaps the weed or a small drop of pesticide strategically placed is all that is required to get the job done. However, identifying the weeds in a real-life situation with many unknowns is much more difficult.
Different Algorithms for Different Weeds
The steps required to train such a weed-detecting robot are the already familiar ones for these algorithms. First, you need to acquire data. In this case, this is essentially a set of high-quality images of agricultural fields, either in the visual range or at different wavelengths with multispectral cameras. These images then undergo pre-processing, such as removing background noise, enhancing relevant features, and potentially using vegetation indices or other indicators.
“To train an effective model for weed detection and evaluate the performance of the learned model, the dataset is usually divided into training data and test data,” write the authors of a 2022 review on existing technologies. “The training set is used to train and tune the hyper-parameters of the model. The test set is used to provide an unbiased evaluation of a final model fitted by the training set. Sometimes, we also need to split a validation set from the training samples to observe the performance of the learned model to help in the final model selection.”
Afterwards, features that can be used to distinguish between crops and weeds are extracted. These features can be based on color, shape, texture, or relevant spectral properties. For instance, weeds may look relatively similar to crops, but they may have a higher (or lower) reflectivity, which can be detected (it is particularly here that multispectral cameras are useful, as they can show features not visible to the human eye). Subsequently, various ML algorithms are used to classify the extracted features into weed and crop categories.
Common algorithms include k-Nearest Neighbors (KNN), Support Vector Machines (SVM), and Convolutional Neural Networks (CNNs). Each algorithm has its strengths, with CNNs particularly excelling in image recognition tasks due to their ability to automatically learn and extract relevant features from raw images. The selected algorithms are trained – commonly using labelled datasets – and then validated and tested.
The major challenge is that even if such a model works, it will likely only work for the specific instance in which it was trained. Not all weeds are alike and not all crops are alike, so you cannot just train a model for every weed and every crop, you need to do it with specific plants in mind. Several datasets are already publicly available for training algorithms, but there is no “silver bullet” algorithm that works best in all cases.
A recent study from December 2023 summarized the challenge thusly:
“The key challenge in crop-weed classification for annotation-based techniques is the construction of the classification model and the optimization of the model parameters. The classifier model, like any other image classification issue, is created for specific applications, and its parameters must be fine-tuned and optimized. Classifier optimization necessitates the use of several algorithms in order to attain a high classification rate while minimizing false positives and data overfitting.”
“The biggest problem is how the technology is used, followed by the ways used owing to crop and weed morphology. As a result, determining which techniques are preferable on an “apple-to-apple” basis is extremely challenging.”
This Is Already Happening
Automated weeding is more than just a good idea. Several such systems have already been developed in university settings and are starting to become commercialized. Autoweeders (armed with either lasers or poison) are routinely achieving a weed elimination rate of around 70%, and while they still have to be followed by a “manual” verification, this enables farmers to reduce the use of pesticides and make more sustainable decisions.
In addition, ML models can be used with either autonomous robots or IoT devices to create fully integrated weed management systems. For instance, you could continuously monitor fields and detect weeds, eliminating them without human intervention. Another approach would be to use drones to monitor larger areas and detect weeds and then have a separate tool destroy the weeds.
There are still important challenges, particularly when it comes to scaling the approach to different types of crops and different types of weeds. This may need to be done on a case-by-case basis, which will be time and resource-consuming. Ultimately, however, automated weeding has come a long way in only a few years and improvements in imaging technology, sensors, and machine learning algorithms are all pushing the boundaries of automated weeders.
Future iterations of these technologies could integrate more sophisticated AI models capable of learning and adapting to new weed species and evolving field conditions. Additionally, as the approach becomes implemented in real situations, collaborative efforts between researchers and farmers can also make automated weeders more robust and adaptable.
For millennia, agriculture has been a cornerstone of our society – and will continue to be so. Feeding the world sustainably is no easy feat, but we have new “weapons” at our disposal. By leveraging these technologies, we can enhance crop yields, reduce farming’s environmental impact, and contribute to the greener future of agriculture.
The post Agriculture 5.0: Combating Weeds Using Machine Learning and Robotics originally appeared on the HLFF SciLogs blog.