Almost every protein known to science: AlphaFold’s revolution is right around the corner
Andrei Mihai
Imagine you’re the best chess player on the planet. You’ve conquered it all, you’re even playing a way that’s hard for others to understand – so you move on to bigger things.
You start mastering the ancient game of Go, which is 1 million trillion trillion trillion trillion more complex than chess. No one really believes in you, and yet you do it. Out of nowhere, and in startling fashion, you beat the Go world champion 4-1, producing a few stunning strategic innovations along the way.
So again, you move on to different things. Starcraft – that’s easy. A bunch of Atari games – no sweat. No logic game holds an opponent for you. So what’s left? Well, using your skills to make a positive impact in the world is left – and that’s exactly what AlphaFold has done.

From games to protein
The above story isn’t made up, it’s very real. It’s just that the main hero isn’t exactly human, it’s AlphaGo – and AlphaStarcraft and all its AI “cousins” developed by DeepMind, a British artificial intelligence subsidiary of Alphabet, Google’s parent company.
This is where AlphaFold, the latest iteration of the AI program, enters the stage. AlphaFold performs predictions of protein structure, a problem that’s as challenging as it is important. The 3D structure of proteins is crucial to understanding their function; similarly, if you want a protein that can perform a certain task, you need to have a good idea about what shape it should have.
However, the “protein folding problem”, as it’s sometimes called, is extremely challenging. It involves understanding not just the thermodynamics of protein structures, but also the interatomic forces that determine the stable structure of proteins – and every time you need to simulate something down to the atomic level, things are bound to get tricky.
Researchers have been struggling with this for decades, and even with advanced algorithms and a qualified team, it’s still such a major challenge. This is where AlphaFold enters the stage. No focusing on games, AlphaFold works to solve this very pragmatic problem, Pushmeet Kohli PhD, Head of AI for Science, DeepMind, told me in an email.
“Our goal has always been to use AI to accelerate scientific endeavour and better understand the world around us. The first few years of DeepMind, including our work on AlphaGo, was focused on making progress on fundamental competencies of AI systems. Our work on protein folding began in 2016 after AlphaGo.”
Kohli continued, “The ‘protein folding problem’ seemed well suited to AI due to the wealth of data already available via the Protein Data Bank, and the biennial CASP competition, regarded as the gold standard for assessing predictive techniques, which would allow us to test the accuracy of our predictions against real experimental data in a rigorous, blind assessment. “
AlphaFold steps up
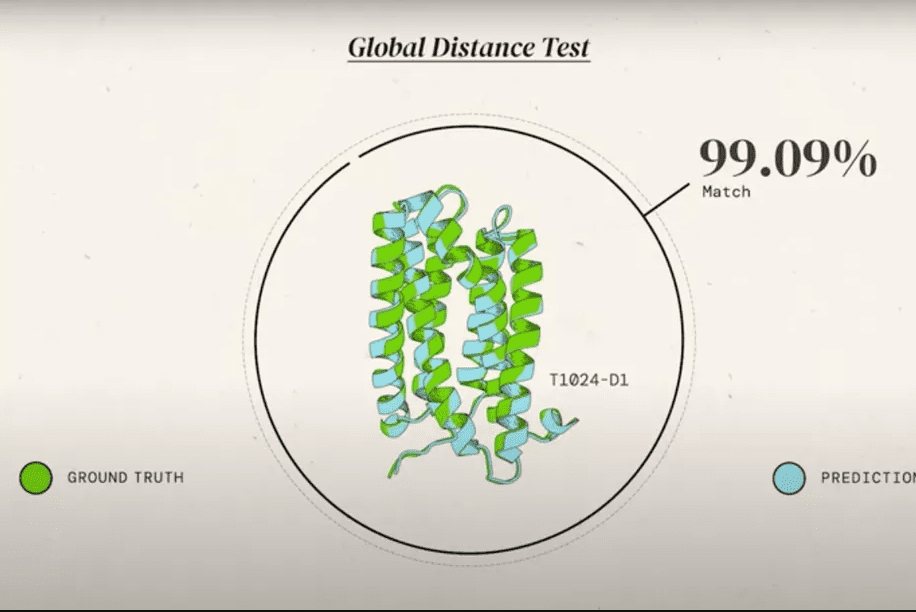
AlphaFold (or rather, AlphaFold 2, the second version of the algorithm) first took the stage in 2018, at the Critical Assessment of protein Structure Prediction (CASP).
CASP is an event where all researchers can participate and are offered a chance to test their protein folding prediction algorithms. It’s a bit like the World Cup of the field, and it works like this.
CASP organizers decide on some protein structures that have only recently been experimentally determined but have not been published yet. The participating teams are asked to compete in a double-blind system (neither the predictors nor the organizers know these structures) and predict the shape of these proteins.
At CASP13, in 2018, AlphaFold outperformed all other competitors. For DeepMind, this meant they were on the right track. Two years later, at CASP14, they were even more successful, surpassing every competing algorithm by far.
“We didn’t know exactly how successful AlphaFold was until we saw the CASP13 results as we could never be fully sure that there wasn’t an error somewhere in the system or a built in bias that would only be shown up in a blind test,” Kohli reported. “Our initial reaction when we saw that AlphaFold achieved the highest accuracy among entries was one of excitement and happiness that our original hypothesis that AI would accelerate scientific progress was true. After our continued success at CASP14, we then turned our attention to how we could give the wider scientific community access to AlphaFold and its predictions.”
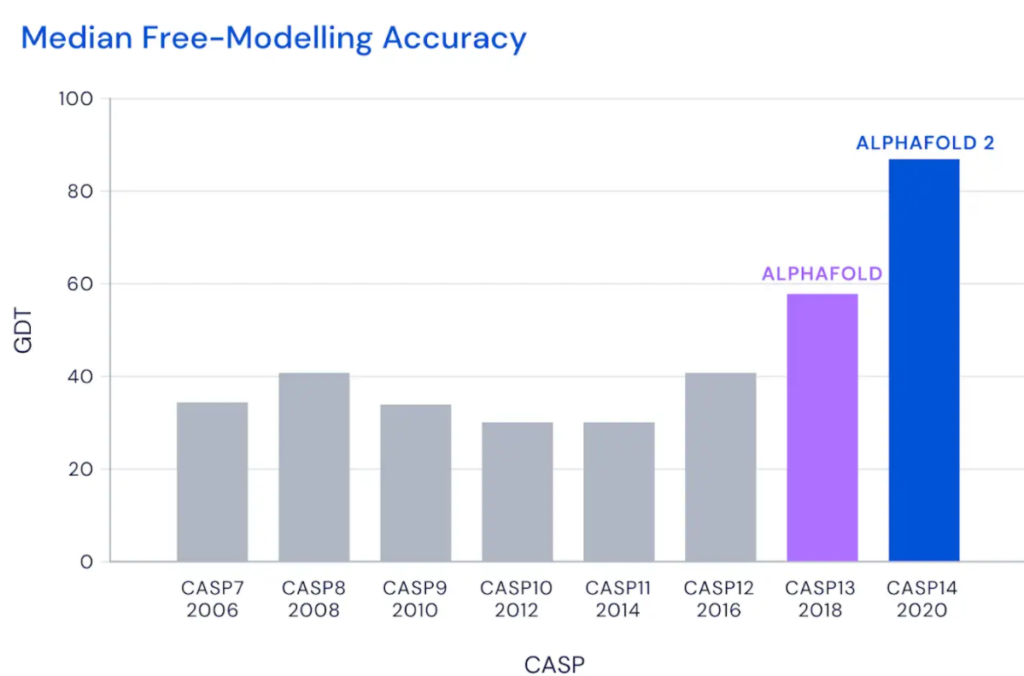
Every protein known to science
As it turns out, this is still only the beginning for AlphaFold. Over the next few months, DeepMind says it will release the folding structure of some 100 million proteins – almost all the proteins known to science.
“Over the coming months we plan to vastly expand the coverage of the database to almost every sequenced protein known to science, with over 100 million structures covering most of the UniProt reference database. We also plan to set up a web interface so researchers can easily predict the structure of any sequence using AlphaFold.”
The company also announced they will publish the full details of that tool and released its source code. For Kohli, this is particularly exciting.
“We have always been determined to put the power of AlphaFold in the hands of scientists, so we are excited to see how the community will use the database to accelerate their work, and what applications are developed in the coming months and years. The database, combined with AlphaFold’s capabilities, provide structural biologists with powerful new tools for examining a protein’s three-dimensional structure and offer a treasure trove of data that could unlock future advances and herald a new era for AI-enabled biology.”
AI is just getting started
It’s often the case that the impact of AI and deep learning are overstated, but this is not the case.
The importance of having access to this type of protein folding library is tremendous. Much like the Human Genome Project drove rapid and spectacular advancements in the field of medicine, a proteome library could usher in a new revolution in medicine (there are other applications besides medicine, but this seems to be the main focus).
For Kohli and DeepMind, this means they’re just getting started.
“Over the next decade, we want to continue to apply AI to better understand the world around us and accelerate progress on some of our most fundamental and fascinating scientific challenges. AlphaFold is our first significant milestone in demonstrating this but we are also making good progress on problems related to nuclear fusion, quantum chemistry and weather prediction.”
Also, unlike many technological developments, which are initially only applicable in the developed world, this one can have a global impact early on and can be particularly useful for the developing world.
“AlphaFold is already being used by partners such as the Drugs for Neglected Diseases Initiative (DNDi), which has advanced their research into life-saving cures for diseases that disproportionately affect the poorer parts of the world, and the Centre for Enzyme Innovation (CEI) is using AlphaFold to help engineer faster enzymes for recycling some of our most polluting single-use plastics.”
Kohli went on, “Ultimately, we hope this advancement will continue to open up new avenues of scientific inquiry that will advance our understanding of everything from problems relating to antibiotic resistance and environmental sustainability, as well as expanding our understanding of living systems beyond the human proteome.”
A special thanks goes to Pushmeet Kohli at DeepMind for providing these insights, and one can’t help but wonder what’s next. For now, at least, AlphaFold is done playing games – it’s ready to take on real challenges, and the results are already thrilling.
The post Almost every protein known to science: AlphaFold’s revolution is right around the corner originally appeared on the HLFF SciLogs blog.